Supporting foodborne outbreak investigations: A review of the use of whole genome sequencing and emerging technologies
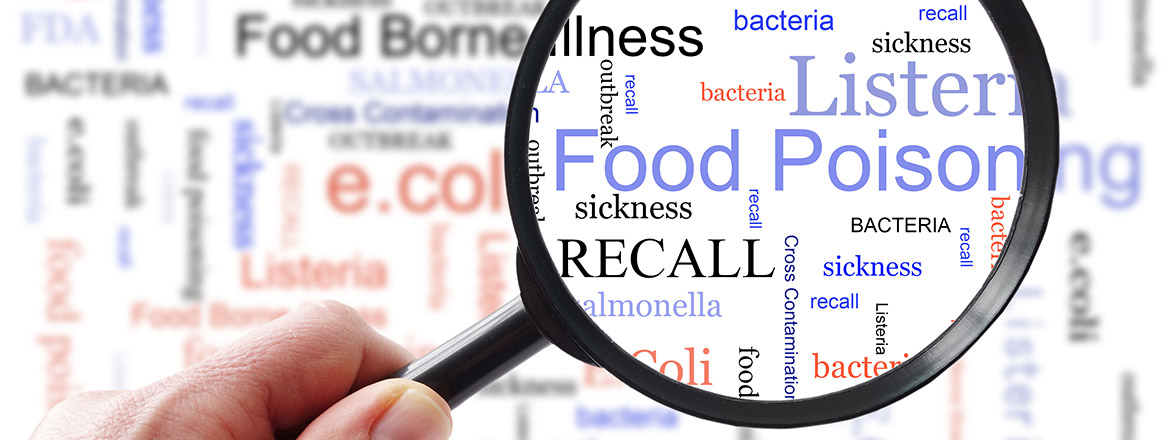
Key Messages |
A paradigm shift in foodborne outbreak investigations has occurred with the emergence of whole genome sequencing (WGS). WGS provides these improvements over traditional laboratory methods:
|
Introduction
Outbreaks of foodborne diseases continue to affect populations across Canada and worldwide, resulting in significant adverse health1 and economic impacts.2 The molecular landscape in foodborne disease (FBD) outbreak investigations is rapidly changing from traditional molecular subtyping to WGS methods.3 Globalized food systems are leading to larger and more complex outbreaks that are challenging to investigate.4 Modern outbreak investigations require multiple and interdisciplinary stakeholders working together to identify affected individuals and common exposures, isolate pathogens, identify source(s), contain the outbreak, and communicate investigative information to health partners and the public.
This paper is the first in a series that provides guidance for the collaborative investigation of foodborne outbreaks. The focus of this first document is a review of WGS and other emerging technologies in foodborne outbreak investigations. Subsequent papers will focus on the roles and responsibilities of different groups and agencies involved in foodborne outbreak investigations, particularly those held by environmental public health professionals.
Methodology
A semi-systematic review5 was completed to explore how emerging technologies, primarily WGS, can be used to detect and investigate FBD outbreaks. Scholarly, grey literature, and government websites were searched for information on the use of WGS and other emerging technologies, in FBD outbreaks, using PubMed, CINAHL, Food Science Source, Google Scholar, and Google databases. Search strategies were adjusted for each platform’s specific format.
Relevant English-language results were collected from January 2016 to June 2022. Complete search terms and the full list of results are available upon request. This semi-systematic review was scoped to reviews related to WGS, while briefly covering other technologies and data sources supporting FBD investigations. The literature was assessed by a single reviewer, and the results were synthesized narratively and were subjected to internal and external review.
Results
Several reviews identified the ability of WGS molecular tools to support foodborne outbreak investigations, particularly multi-jurisdictionally within Canada and the United States, as well as globally. Across the reviews, WGS demonstrated unparalleled resolution (amount and specificity of isolates genetic material) and discriminatory (ability to differentiate and link isolates) powers surpassing traditional molecular methods.3,6-23 An overview of the key findings for each review are presented in Table 1. Two additional promising emerging technologies that support data analysis and collection of genetic material respectively, were identified: machine learning22,24-26 and biosensors.27-31 Further, metagenomics15,32-34 and consumer purchase data23,35 are good examples of metadata available to support FBD outbreak investigations. Predominantly used for bacterial isolates, WGS is beginning to be used for other pathogens including fungus and parasites,36 as well as viruses.37,38 As the emerging gold standard at the national (Canada and US) and international levels for identification and comparison between foodborne pathogens, this review will focus on WGS.
WGS represents an all-in-one approach to microbial identification and discrimination, replacing a plethora of traditional testing methods, such as Pulsed-field Gel Electrophoresis (PFGE) and serotyping.9,12,39 WGS has been shown to support foodborne outbreak investigations, epidemiological follow-up, traceback, surveillance, and source attribution.15,17 WGS is a molecular tool used by industry and public health to inform risk assessments and help shape policy.20 Further, WGS allows for expansive exploration of sources during outbreak investigations, encapsulating an interdisciplinary and One Health approach to foodborne outbreak investigations.17,39 The emergence of WGS strengthens the call for increased multidisciplinary workforces to be capable of predicting and adapting to changing food safety risks in order to respond effectively to foodborne outbreaks.20
WGS methods
The two main approaches to WGS, base-by-base (single-nucleotide polymorphism [SNP] analysis) and gene-to-gene (multilocus sequence typing [MLST]) are summarized in Table 2. Detailed descriptions of these sequencing methods are described elsewhere.3,6,8,13,21,22
SNP analysis uses almost all the genetic information from a genome/strain, and thus theoretically provides the highest level of precision available for the reconstruction of strain phylogeny.6 However, core genome MLST (cgMLST) is currently the most commonly used method for outbreak investigations,9,12 and it provides the best option for data sharing between agencies and across borders.22 Both methods provide strong resolution and discrimination for isolates as compared to traditional methods.
Generally, the higher the genetic similarity between isolates means that they are more closely related (see Table 4 for an example of WGS genetic comparison for SNP method). For MLST, relatedness is determined by comparing gene sequences where isolates that have matching sequence types (ST) are defined as clonal, meaning they share a common ancestor.3 The relatedness of isolates can be inferred by the number of STs in common. It is important to remember that foodborne pathogens often have very short generation times under ideal growth conditions. Therefore investigators can anticipate small amounts of genetic variation in clinical, food, and environmental isolates during an outbreak investigation.6
The interpretation of variation between isolates requires training, quality reference banks, and access to specialists with bioinformatic skills who can run complex computations and follow best practices for data interpretation.3,5,7,12 Regardless of the WGS method, successful analysis requires well-developed bioinformatic workflow pipelines.21 Strong bioinformatic pipelines start with data quality control, where segments with poor read quality or those that do not carry biological information are excluded.12,21 Numerous web-based tools are available for public health, laboratory, and research to organize and analyse WGS data, reducing the costs associated with complex computers and need for on-site bioinformatic specialists.12 However, WGS sequencing without established pipelines, high-quality references, and strong data analysis can result in false positives and is open to gross misinterpretation.7 For example, a recent study of burger meat initially attributed 3–5% of the DNA to monkeys, where further analysis clarified it was from cattle.22
WGS in outbreak investigations and surveillance
In conjunction with traditional epidemiological and field investigations, WGS provides unparalleled clarity of associations between sources, suspect food, and clinical isolates.3,6-8,10,23,40 Specifically, WGS can be used to:
- develop specific and sensitive case definitions for outbreaks;
- shed light on pathogen introduction, harborage, cross contamination, source attribution, and temporal and geographic distribution;6
- construct evolutionary relationships of isolates in a foodborne outbreak investigation;3 and
- detect a higher number of small or diffuse outbreaks than traditional methods, demonstrating improvements in detection of temporal and spatial clusters.3,7
Recent examples of the use of WGS in FBD investigations are presented in Table 3.
Despite the benefits of WGS, it is critical that WGS and other emerging technologies be used in conjunction with epidemiological and field investigations.7,8,17,23 Investigators must query if the WGS results make epidemiological sense8 by taking into consideration clustering by time, geographic location, food history, and exposure.17,23 Public health actions, especially enforcement or recalls that are based on WGS results require strong epidemiological and field investigation links.7,12,23 To improve multinational outbreak investigations across borders and globally, public health, industry, and academia need to harmonize methods, and continue to build and share open-reference databases to address foodborne outbreaks.12
WGS as an all-in-one molecular tool
WGS provides a single analysis method to sequence entire genomes and to identify and characterize pathogens, including typing antimicrobial resistance (AMR) and virulence profiles that is rapid and cost efficient.3,6,22 Further, using the same methodology for clinical, food, and environmental isolates is crucial for matching and interpretation of results.12 Pathogen WGS carry geographic information that can help identify sources.22 Thus, WGS is ideally suited for use in any foodborne outbreak, with particular emphasis on national and international surveillance systems in support of harmonized food safety and public health.6,22
WGS vs. traditional molecular tools
In the early 2010s, with its resolution and discriminatory advantages, WGS began to replace PFGE as the preferred subtyping method.6,15 WGS was initially applied retrospectively to characterize historical isolates to improve past outbreak investigations.6 Importantly, the overall WGS results were concordant with traditional investigation methods,12 thus presenting an opportunity to compare historical and future isolates. Further, WGS has demonstrated better results compared to traditional methods, helping to resolve unidentified outbreaks.12 Lastly, WGS has been shown to overcome problems associated with PFGE over-discrimination sometimes found in L. monocytogenes outbreaks, where cgMLST showed clonal relations between isolates, despite differing PFGE patterns.3
In 2013, PulseNet launched a pilot project in parallel with PFGE-based surveillance for analyzing isolates of L. monocytogenes using WGS.6,15 WGS provided higher resolution and precision than PFGE and, as a result, more outbreaks could be detected and investigated. Further, the benefits of the PulseNet system are that all participating laboratories use the same algorithm(s), molecular standards, and protocols, enabling faster and more efficient comparison of genetic profiles across jurisdictions.3 Equally as important, WGS debunked false outbreak signals from PFGE matching. The pilot study also showed that very small outbreaks with few isolates (e.g., two matched clinical cases) could be then matched to food isolates already sequenced by the US Food and Drug Administration or Food Safety and Inspection Service.6
Recent advancements in technology and bioinformatics allow researchers to generate in silico results for traditional methods from WGS data, permitting the comparison of isolates.3 Current software can now predict the pathogenicity of an organism based on its WGS, which helps with risk assessment and mitigation efforts.3 An area for future research points to the ability to use WGS information to predict phenotypes, accurately determining virulence and other risk factors to support public health and food safety control measures.3
Additional benefits of WGS
Beyond the all-in-one benefits and use in outbreak investigation and surveillance as stated above, WGS offers additional benefits over traditional methods, including source attribution, rapid response, decreased costs, harmonization of data, root cause analysis, and detection of pathogenic factors.
Source attribution
WGS will likely revolutionize microbiological source attribution of sporadic foodborne illness and expand our knowledge of the epidemiology of different infectious diseases.6 WGS can also help investigators distinguish between new and recurrent pathogens in food premises by comparing genetic variation.8
Quicker response and decreased costs
WGS may allow for public health outbreak interventions at earlier stages as well as the identification of more outbreaks,6,8 resulting in earlier elimination of sources and decreasing associated health and economic costs.20 A recent economic evaluation of PulseNet, which uses WGS, estimated that the program prevents at least 270,000 foodborne illnesses and leads to savings of over $500 million in medical and productivity costs in the US each year.2 While detection of more outbreaks can provide new insights on sources and pathogenic spread,20 increasing outbreak investigations could tax the public health system’s ability to respond and mitigate risk due to increased resource demand.8
Harmonization and interpretation of WGS data
Harmonization and standardization of WGS methods and interpretation across borders and sectors (human, animal, environmental, and food) is needed to allow data sharing and to ensure consistent responses.3,21,22 Successful harmonization requires a globally accessible database and rapid uploading of data for real-time analysis.39
There are two basic ways to achieve this harmonization, namely through validation of different methods to demonstrate equivalent results, e.g., Global Microbial Identifier (GMI), or the use of standard operating procedures (SOP) as has been done with PulseNet and GenomeTrakr.21 Alongside PulseNet USA, GenomeTrakr partners include reference laboratories around the world, including Canada.22,41 A retrospective study of Shigella sonnei through PulseNet Latin America and Caribbean (a sub-set of PulseNet International) successfully demonstrated the use of WGS to contextualize local outbreaks and to identify a new global lineage, thus highlighting the globalization of FBD outbreaks.4
Root cause analysis
Another reported benefit of WGS is its ability for use by investigators and the food industry for root-cause analysis. WGS resolution and discrimination, including detailed phylogenetic trees, support accurate traceback and trace forward of pathogens in foods, as well as the narrowing of sources of contamination, permitting implementation of effective control measures.8,17 The realization of the potential of WGS requires a tripartite (government, industry, and academia) multidisciplinary approach involving investment in training and testing capacities.20
Detection of pathogenic factors
A final benefit of WGS is for characterizing potential virulence factors and antimicrobial resistance of isolates for improving outbreak response as well as for patient treatment.3,22,42 Doughman et al.42 outlines the potential for WGS to explain virulence factors of Salmonella strains, thus helping to determine why some isolates are more likely to cause disease. WGS can also improve understanding of sporadic and continuous Listeria contamination, residence in biofilms, and tracing in processing facilities.43
Emerging metadata trends – metagenomics, biosensors, and consumer information
Emerging metadata that includes WGS metagenomics, data from industry biosensors, and consumer data, represent opportunities to support the identification and investigation of foodborne outbreaks. Examples of platforms using big data to support FBD investigations are shown in Table 5. As discussed above, outbreak metadata include the who, what, when, and where associated with each sample and isolate processed. Biosensors that can help industry measure food quality and safety parameters, including identification of pathogens, are emerging for use in the food industry.27,44,45 Consumer use of loyalty cards, as well as routine posts of eating habits and suspect foodborne illnesses across social media platforms, presents another rich source of data for investigators.23 Consumer data was shown to be particularly useful in outbreaks caused by agents with a long incubation time or by several different products, products with long shelf lives, low brand recognition, or those representing subsets of foods that are very commonly consumed.35
Machine learning
The availability of vast amounts of genomic, phenotypic, and meta-data for pathogenic organisms has led to the development of several algorithms and databases that are remarkably accurate at predicting isolates, host specificity, virulence, and AMR.15,22 EpiDMS46 is one example of emerging data management and analytics tools available to support epidemic investigations. Machine learning methods recognize patterns in datasets and use this information to build models, to identify the genetic variations in isolates, and to help identify potential sources.24 Recent studies have looked at algorithms capable of predicting pathogens when the pathogen is unknown or not tested, based on temporal, spatial, food history, and symptomatology, with some success.26
Limitations and challenges of WGS
The biggest challenge for WGS is in interpreting the rates and amount of genetic variation over time among microorganisms. Generally, the more similar the genome is between isolates, the more closely they are related. Determining similarity is organism specific and dependent on the size and conservation of the genome. An example of this point is Listeria, which has a smaller and more conserved genome than Salmonella, E. coli, and Campylobacter.47 The longer an organism persists in the environment or host, the more genetic variation is anticipated.17,48 Interpretation of WGS results are complicated by differences in rates of genetic variation between microbiological species and rates of variation in different environments.22 This is illustrated in a study by Petronella et al48 that found the amount of genetic drift varied significantly for four common public health pathogens when measuring mutations over time in cultured samples. These genetic variations make developing tools and references for genetic relatedness difficult and organism specific. An example of this is shown in Table 4.
Other concerns with the implementation and analysis of WGS for foodborne outbreak investigations include standardization, consistency, political will, funding, and sharing of sensitive metadata, especially with international partners.12 Of note is that collection and testing of isolates provides large volumes of metadata that include confidential and sensitive data such as patient personal and health information, identities of food processors and other information, all of which require strong policies around the collection, storage, and sharing of information.8 Some of these concerns have been effectively addressed by GenomeTrakr, which sets the minimum fields for metadata related to isolates in the system (who, what, when, where), providing enough data for course tracking but not implicating individuals or specific facilities. Such metadata is kept confidential.39 Public health and health care policies need to be developed that encourage the collection and sharing of patient, food, and environmental samples to ensure WGS can be performed and data readily shared.
Lastly, clinical and some public health laboratories are switching to rapid, non-culture tests, e.g. MALDI-TOF,49 meaning there may be fewer (or no) isolates from patients with foodborne illnesses. This highlights a critical limitation in using WGS for foodborne outbreak investigations as the technique requires sufficient isolates from cases and foods for sequence.3
Summary
The emergence of WGS represents an all-in-one approach to microbial identification and discrimination, capable of predicting and adapting to changing food safety risks. WGS supports foodborne outbreak investigations, epidemiological follow-up, traceback, surveillance, and source attribution. WGS represents a paradigm shift in FBD outbreak information. Due to declining costs, user-friendly software applications, unparalleled resolution, enhanced discrimination, an all-in-one approach, and value-added secondary analysis of virulence and AMR, WGS will continue to replace traditional molecular methods.3 This transition represents opportunities for enhanced sharing of rapid isolate information in a multidisciplinary and international food safety environment. WGS, combined with rapidly evolving sources of metadata (genomics and consumer reported), represents an opportunity to supplement traditional investigative methods and increase effectiveness of surveillance and response, from the local to international outbreak investigation levels. Public health should continue to enhance the use of WGS in foodborne disease investigations and work with government, laboratory, and industry partners to develop consistent and standardized approaches for the collection of samples, testing, and interpretation of results. Future foodborne disease investigations should look to use WGS and other emerging trends including sources of foodborne metadata and machine learning to enhance investigations.
Acknowledgements
I would like to thank Lorraine McIntyre, Food Safety Specialist from the BC Centre for Disease Control, and Dr. Wendy Pons, Conestoga College ITAL, for lending their expertise and experience as internal and external reviewers respectively.
Glossary
Term |
Definition |
Discrimination |
Ability to differentiate and link isolates based on their genome. |
Harmonization |
Here harmonization is used to describe the consistent collection, testing, and analysis of isolates to allow for data sharing and accurate interpretation. |
Metadata |
Here metadata is used to capture all of the who, what, when, where, data related to FBD investigation samples and isolates. |
Metagenomics |
Study of the entire genetic structure and function of an isolate, typically a microbe. |
Biosensor |
Device consisting of a biological component (such as an enzyme) that reacts with a target substance and an electrochemical or optical component that detects the target analyte. |
Pipeline |
Workflows needed for isolate collection, preparation, and referencing to ensure accurate results. |
Resolution |
Amount and specificity of DNA material provided from a given testing method. |
Traditional molecular methods |
Laboratory methods used prior to WGS, including serotyping and PFGE. |
Table 1. Whole genome sequencing reviews used in the semi-systematic review.
Title |
Author |
Year |
Publication Title |
Use of Whole-Genome Sequencing for Food Safety and Public Health in the United States |
Brown et al.6 |
2019 |
Foodborne Pathog Dis |
Genomic Epidemiology: Whole-Genome-Sequencing—Powered Surveillance and Outbreak Investigation of Foodborne Bacterial Pathogens |
Deng et al.13 |
2016 |
Annual Review of Food Science and Technology |
Novel opportunities for NGS-based one health surveillance of foodborne viruses |
Desdouits et al.18 |
2020 |
One Health Outlook |
Significance of whole genome sequencing for surveillance, source attribution and microbial risk assessment of foodborne pathogens |
Franz et al.7 |
2016 |
Current Opinion in Food Science |
Whole Genome Sequencing: Bridging One-Health Surveillance of Foodborne Diseases |
Gerner-Smidt et al.17 |
2019 |
Front Public Health |
Emerging needs and opportunities in foodborne disease detection and prevention: From tools to people |
Hoelzer et al.20 |
2018 |
Food Microbiology |
The use of next generation sequencing for improving food safety: Translation into practice |
Jagadeesan et al.8 |
2019 |
Food Microbiology |
Whole Genome Sequencing: The Impact on Foodborne Outbreak Investigations |
Kovac et al.22 |
2020 |
Reference Module in Food Science |
Whole genome sequencing as a typing tool for foodborne pathogens like Listeria monocytogenes – The way towards global harmonisation and data exchange |
Lüth et al.21 |
2018 |
Trends in Food Science & Technology |
The Benefits of Whole Genome Sequencing for Foodborne Outbreak Investigation from the Perspective of a National Reference Laboratory in a Smaller Country |
Nouws et al.12 |
2020 |
Foods |
Navigating Microbiological Food Safety in the Era of Whole-Genome Sequencing |
Ronholm et al.3 |
2016 |
Clinical Microbiology Reviews |
Advances in foodborne outbreak investigation and source tracking using whole genome sequencing |
Ruppitsch et al.11 |
2019 |
IOP Conference Series: Earth and Environmental Science |
Use of Whole-Genome Sequencing at the Food Safety and Inspection Service to Detect and Investigate Foodborne Illness Outbreaks |
Shaw et al.10 |
2020 |
Food Protection Trends |
Techniques in bacterial strain typing: past, present, and future |
Simar et al.9 |
2021 |
Current opinion in infectious diseases |
Use of Whole Genome Sequencing by the Federal Interagency Collaboration for Genomics for Food and Feed Safety in the United States |
Stevens et al.15 |
2022 |
Journal of food protection |
Phylogenomic Pipeline Validation for Foodborne Pathogen Disease Surveillance |
Timme et al.19 |
2019 |
J Clin Microbiol |
Food safety trends: From globalization of whole genome sequencing to application of new tools to prevent foodborne diseases |
Wang et al.14 |
2016 |
Trends in Food Science & Technology |
Big Data for Infectious Diseases Surveillance and the Potential Contribution to the Investigation of Foodborne Disease in Canada: An Overview and Discussion Paper |
Waldner23 |
2017 |
National Collaborating Centre for Infectious Diseaseas |
Advances in typing and identification of foodborne pathogens |
Wei et al.16 |
2021 |
Current Opinion in Food Science |
Table 2. Features of Whole Genome Sequencing methods for bacterial organisms
NGS Method |
Description |
Use / Advantages |
Limitations |
Base to Base – single nucleotide polymorphism (SNP)6 |
Two types: 1. Reference based 2. Reference-agnostic/k-mer The SNP profiles of all isolates are compared in a pairwise manner and usually displayed in the form of a phylogenetic tree6 |
Highest discriminatory power6,9 Particularly useful when small number of isolates available9 Reference free methods are most reliable in suspected outbreak situations, of where isolates are expected to be relatively similar9 Used by FDA-CFSAN, USDA-FSIS, and many other GenomeTrakr partners6 |
Selection of reference genome paramount to success6,9 |
Gene to gene – multilocus sequence typing (MLST)6 |
Two methods: 1. Core genome cgMLST 2. whole genome wgMLST |
cgMLST is most commonly approach currently used, offering standardization and transferability across labs8,9,12 wgMLST is best option when investigating highly related organisms. Used by PulseNet6 and PulseNet International3 |
High quality references needed for discriminatory power9 Lacking standardized method of classification9 Requires high level of bioinformatic expertise9 |
Table 3. Examples of the use of WGS in FBD investigations
Title |
Year |
Author |
Publication Title |
Genetic diversity of Listeria monocytogenes strains contaminating food and food producing environment as single based sample in Italy (retrospective study) |
2022 |
Acciari et al.50 |
International Journal of Food Microbiology |
Whole genome sequencing of Shigella sonnei through PulseNet Latin America and Caribbean: advancing global surveillance of foodborne illnesses |
2017 |
Baker et al.4 |
Clinical Microbiology and Infection |
Highly Pathogenic Clone of Shiga Toxin-Producing Escherichia coli O157:H7, England and Wales |
2018 |
Byrne et al.51 |
Emerging Infectious Diseases |
Characterization of Emetic and Diarrheal Bacillus cereus Strains From a 2016 Foodborne Outbreak Using Whole-Genome Sequencing: Addressing the Microbiological, Epidemiological, and Bioinformatic Challenges |
2019 |
Carroll et al.52 |
Frontiers in Microbiology |
Whole-Genome Sequencing of Salmonella Mississippi and Typhimurium Definitive Type 160, Australia and New Zealand |
2019 |
Ford et al.53 |
Emerging Infectious Diseases |
Investigation of Outbreaks of Salmonella enterica Serovar Typhimurium and Its Monophasic Variants Using Whole-Genome Sequencing, Denmark |
2017 |
Gymoese et al.54 |
Emerging Infectious Diseases |
Large Nationwide Outbreak of Invasive Listeriosis Associated with Blood Sausage, Germany, 2018–2019 |
2020 |
Halbedel et al.55 |
Emerging Infectious Diseases |
Ability of Whole-Genome Sequencing to Refine a Salmonella I 4,[5],12:i:-Cluster in New York State and Detect a Multistate Outbreak Linked to Raw Poultry |
2021 |
Huth et al.56 |
Food Protection Trends |
Whole-Genome Sequencing to Detect Numerous Campylobacter jejuni Outbreaks and Match Patient Isolates to Sources, Denmark, 2015–2017 |
2020 |
Joensen et al.57 |
Emerging Infectious Diseases |
Nationwide outbreak of invasive listeriosis associated with consumption of meat products in health care facilities, Germany, 2014–2019 |
2021 |
Lachmann et al.58 |
Clinical Microbiology and Infection |
Whole-Genome Analysis of Salmonella enterica Serovar Enteritidis Isolates in Outbreak Linked to Online Food Delivery, Shenzhen, China, 2018 |
2020 |
Min et al.59 |
Emerging Infectious Diseases |
Real-Time Whole-Genome Sequencing for Surveillance of Listeria monocytogenes, France |
2017 |
Moura et al.60 |
Emerging Infectious Diseases |
Application of Whole‐Genome Sequences and Machine Learning in Source Attribution of Salmonella Typhimurium |
2020 |
Munck et al.24 |
Risk Analysis: An International Journal |
Use of whole-genome sequencing for public health intervention: outbreak investigation of a cluster of cases of salmonella foodborne illness in England, 2016 |
2018 |
Olufon et al.61 |
The Lancet |
Genetic characterization of norovirus GII.4 variants circulating in Canada using a metagenomic technique |
2018 |
Petronella et al.37 |
BMC Infectious Diseases |
Application of whole-genome sequencing for norovirus outbreak tracking and surveillance efforts in Orange County, CA |
2021 |
Silva et al.38 |
Food Microbiology |
Evaluation of WGS based approaches for investigating a food-borne outbreak caused by Salmonella enterica serovar Derby in Germany |
2018 |
Simon et al.62 |
Food Microbiology |
Escherichia coli O103 outbreak associated with minced celery among hospitalized individuals in Victoria, British Columbia, 2021 |
2022 |
Smith et al.63 |
Canada Communicable Disease Report |
Genome-wide networks reveal emergence of epidemic strains of Salmonella Enteritidis |
2022 |
Svahn et al.64 |
International Journal of Infectious Diseases |
Outbreak of Reading in persons of Eastern Mediterranean origin in Canada, 2014–2015 |
2017 |
Tanguay et al.65 |
Canada Communicable Disease Report |
Linking Epidemiology and Whole-Genome Sequencing to Investigate Salmonella Outbreak, Massachusetts, USA, 2018 |
2020 |
Vaughn et al.66 |
Emerging Infectious Diseases |
Phylogenetic structure of Salmonella Enteritidis provides context for a foodborne outbreak in Peru |
2020 |
Willi et al.67 |
Scientific Reports |
Table 4. Example of determining genetic relatedness using SNP6
Level of Relatedness |
Allele differences |
Interpretation |
Closely |
0–20 |
Typical in point source outbreaks, with new organism. |
Not clearly related or Unrelated |
20–50 |
Difficult to interpret, often seen in zoonotic outbreaks. |
Unrelated |
>50–100 |
Unrelated for point source outbreaks. Can see this level of difference in polyclonal outbreaks with multiple pathogen strains, or in organisms that persist in an environment for an extended period. |
Table 5. Examples of platforms using big data to support FBD investigations
Platform Name |
Country |
Link |
Integrated Rapid Infectious Disease Analysis (IRIDA)68 |
Canada |
|
GenomeTrakr41 |
United States |
https://www.fda.gov/food/whole-genome-sequencing-wgs-program/genometrakr-network |
PulseNet69 |
United States and International |
|
HealthMap Foodborne Dashboard70 |
United States |
References
- Guldimann C, Johler S. An introduction to current trends in foodborne pathogens and diseases. Curr Clin Micro Rpt. 2018 Apr;5(2):83-7. Available from: https://link.springer.com/article/10.1007/s40588-018-0093-y.
- Scharff RL, Besser J, Sharp DJ, Jones TF, Peter G-S, Hedberg CW. An economic evaluation of PulseNet: a network for foodborne disease surveillance. Am J Prev Med. 2016 May;50(5):S66-S73. Available from: https://doi.org/10.1016/j.amepre.2015.09.018.
- Ronholm J, Nasheri N, Petronella N, Pagotto F. Navigating microbiological food safety in the era of whole-genome sequencing. Clin Microbiol Rev. 2016 Oct;29(4):837-57. Available from: https://doi.org/10.1128/cmr.00056-16.
- Baker KS, Campos J, Pichel M, Della Gaspera A, Duarte-Martínez F, Campos-Chacón E, et al. Whole genome sequencing of Shigella sonnei through PulseNet Latin America and Caribbean: advancing global surveillance of foodborne illnesses. Clin Microbiol Infect. 2017 Nov;23(11):845-53. Available from: https://doi.org/10.1016/j.cmi.2017.03.021.
- Eykelbosh A, Fong D. Conducting a literature search & semi- systematic review: the NCCEH approach. Vancouver, BC: National Collaborating Centre for Environmental Health; 2017. Available from: https://ncceh.ca/sites/default/files/Conducting_Semi_Systematic_Review_NCCEH_Approach-Eykelbosh-Fong.pdf.
- Brown E, Dessai U, McGarry S, Gerner-Smidt P. Use of whole-genome sequencing for food safety and public health in the United States. Foodborne Pathog Dis. 2019 Jul;16(7):441-50. Available from: https://doi.org/10.1089%2Ffpd.2019.2662.
- Franz E, Gras LM, Dallman T. Significance of whole genome sequencing for surveillance, source attribution and microbial risk assessment of foodborne pathogens. Curr Opin Food Sci. 2016 Apr;8:74-9. Available from: https://doi.org/10.1016/j.cofs.2016.04.004.
- Jagadeesan B, Gerner-Smidt P, Allard MW, Leuillet S, Winkler A, Xiao Y, et al. The use of next generation sequencing for improving food safety: translation into practice. Food Microbiol. 2019 Jun;79:96-115. Available from: https://doi.org/10.1016/j.fm.2018.11.005.
- Simar SR, Hanson BM, Arias CA. Techniques in bacterial strain typing: past, present, and future. Curr Opin Infect Dis. 2021 Aug;34(4):339-45. Available from: https://doi.org/10.1097/qco.0000000000000743.
- Shaw SL, Esteban JE, Kissler BW, Freiman JL, Tillman GE. Use of whole-genome sequencing at the food safety and inspection service to detect and investigate foodborne illness outbreaks. Food Prot Trends. 2020 Jul;40(4):268-70. Available from: https://doi.org/10.1089%2Ffpd.2019.2662.
- Ruppitsch W, Pietzka A, Cabal A, Chakeri A, Schmid D, Lakicevic B, et al. Advances in foodborne outbreak investigation and source tracking using whole genome sequencing. IOP Conference Series: Earth and Environmental Science. 2019 Sep;333(1):012010. Available from: https://iopscience.iop.org/article/10.1088/1755-1315/333/1/012010.
- Nouws S, Bogaerts B, Verhaegen B, Denayer S, Crombé F, Rauw KD, et al. The benefits of whole genome sequencing for foodborne outbreak investigation from the perspective of a national reference laboratory in a smaller country. Foods. 2020 Aug;9(1030):1030. Available from: https://doi.org/10.3390/foods9081030.
- Deng X, den Bakker H, Hendriksen R. Genomic epidemiology: whole-genome-sequencing—powered surveillance and outbreak investigation of foodborne bacterial pathogens. Ann Rev Food Sci Technol. 2016 Apr;7. Available from: https://doi.org/10.1146/annurev-food-041715-033259.
- Wang S, Weller D, Falardeau J, Strawn LK, Mardones FO, Adell AD, et al. Food safety trends: from globalization of whole genome sequencing to application of new tools to prevent foodborne diseases. Trends Food Sci Technol. 2016 Nov;57(Part A):188-98. Available from: https://doi.org/10.1016/j.tifs.2016.09.016.
- Stevens EL, Carleton HA, Beal J, Tillman GE, Lindsey RL, Lauer AC, et al. Use of whole genome sequencing by the federal interagency collaboration for genomics for food and feed safety in the United States. J Food Prot. 2022 May;85(5):755-72. Available from: https://doi.org/10.4315/jfp-21-437.
- Wei X, Zhao X. Advances in typing and identification of foodborne pathogens. Curr Opin Food Sci. 2021 Feb;37:52-7. Available from: https://doi.org/10.1016/j.cofs.2020.09.002.
- Gerner-Smidt P, Besser J, Concepción-Acevedo J, Folster JP, Huffman J, Joseph LA, et al. Whole genome sequencing: bridging one-health surveillance of foodborne diseases. Front Public Health. 2019 Jun;7:172. Available from: https://doi.org/10.3389/fpubh.2019.00172.
- Desdouits M, de Graaf M, Strubbia S, Oude Munnink BB, Kroneman A, Le Guyader FS, et al. Novel opportunities for NGS-based one health surveillance of foodborne viruses. One Health Outlook. 2020 Jun;2:14. Available from: https://onehealthoutlook.biomedcentral.com/articles/10.1186/s42522-020-00015-6.
- Timme RE, Strain E, Baugher JD, Davis S, Gonzalez-Escalona N, Sanchez Leon M, et al. Phylogenomic pipeline validation for foodborne pathogen disease surveillance. J Clin Microbiol. 2019 Apr;57(5). Available from: https://doi.org/10.1128/JCM.01816-18.
- Hoelzer K, Moreno Switt AI, Wiedmann M, Boor KJ. Emerging needs and opportunities in foodborne disease detection and prevention: from tools to people. Food Microbiol. 2018 Oct;75:65-71. Available from: https://doi.org/10.1016/j.fm.2017.07.006.
- Lüth S, Kleta S, Al Dahouk S. Whole genome sequencing as a typing tool for foodborne pathogens like Listeria monocytogenes – the way towards global harmonisation and data exchange. Trends Food Sci Technol. 2018 Mar;73:67-75. Available from: https://doi.org/10.1016/j.tifs.2018.01.008.
- Kovac J, Dudley EG, Nawrocki EM, Yan R, Chung T. 1.09 - Whole genome sequencing: the impact on foodborne outbreak investigations. In: Cifuentes A, editor. Comprehensive Foodomics. Oxford: Elsevier; 2021. p. 147-59. Available from: https://doi.org/10.1016/B978-0-08-100596-5.22697-8.
- Waldner C. Big data for infectious diseases surveillance and the potential contribution to the investigation of foodborne disease in Canada. Winnipeg, MB: University of Manitoba; 2017 May. Available from: https://nccid.ca/wp-content/uploads/sites/2/2017/07/Final-English-Big-Data.pdf.
- Munck N, Njage PMK, Leekitcharoenphon P, Litrup E, Hald T. Application of whole-genome sequences and machine learning in source attribution of salmonella typhimurium. Risk Anal. 2020;40(9):1693-705. Available from: https://onlinelibrary.wiley.com/doi/abs/10.1111/risa.13510.
- Vilne B, Meistere I, Grantiņa-Ieviņa L, Ķibilds J. Machine learning approaches for epidemiological investigations of food-borne disease outbreaks. Front Microbiol. 2019 Aug;10:1722. Available from: https://doi.org/10.3389/fmicb.2019.01722.
- Wang H, Cui W, Guo Y, Du Y, Zhou Y. Machine learning prediction of foodborne disease pathogens: algorithm development and validation study. JMIR Med Inform. 2021 Jan;9(1):e24924. Available from: https://doi.org/10.2196/24924.
- Pampoukis G, Lytou AE, Argyri AA, Panagou EZ, Nychas GE. Recent advances and applications of rapid microbial assessment from a food safety perspective. Sensors. 2022 Apr;22(7). Available from: https://doi.org/10.3390/s22072800.
- Neethirajan S, Ahmed SR, Chand R, Buozis J, Nagy É. Recent advances in biosensor development for foodborne virus detection. Nanotheranostics. 2017 Jul;1(3):272-95. Available from: https://doi.org/10.7150/ntno.20301.
- Sharma A, Khan R, Catanante G, Sherazi TA, Bhand S, Hayat A, et al. Designed strategies for fluorescence-based biosensors for the detection of mycotoxins. Toxins. 2018 May;10(5). Available from: https://doi.org/10.3390/toxins10050197.
- Cinti S, Volpe G, Piermarini S, Delibato E, Palleschi G. Electrochemical biosensors for rapid detection of foodborne salmonella: a critical overview. Sensors. 2017 Aug;17(8). Available from: https://doi.org/10.3390/s17081910.
- Huang F, Zhang Y, Lin J, Liu Y. Biosensors coupled with signal amplification technology for the detection of pathogenic bacteria: a review. Biosensors. 2021 Jun;11(6). Available from: https://doi.org/10.3390/bios11060190.
- Buytaers FE, Saltykova A, Denayer S, Verhaegen B, Vanneste K, Roosens NHC, et al. A practical method to implement strain-level metagenomics-based foodborne outbreak investigation and source tracking in routine. Microorganisms. 2020 Aug;8(8):E1191. Available from: https://doi.org/10.3390/microorganisms8081191.
- Carleton HA, Besser J, Williams-Newkirk AJ, Huang A, Trees E, Gerner-Smidt P. Metagenomic approaches for public health surveillance of foodborne infections: opportunities and challenges. Foodborne Pathog Dis. 2019 Jul;16(7):474-9. Available from: https://doi.org/10.1089/fpd.2019.2636.
- Maguire M, Kase JA, Roberson D, Muruvanda T, Brown EW, Allard M, et al. Precision long-read metagenomics sequencing for food safety by detection and assembly of Shiga toxin-producing Escherichia coli in irrigation water. PLoS ONE. 2021 Jan;16(1):1-20. Available from: https://doi.org/10.1371/journal.pone.0245172.
- Møller FT, Mølbak K, Ethelberg S. Analysis of consumer food purchase data used for outbreak investigations, a review. Euro Surveill. 2018 Jun;23(24). Available from: https://doi.org/10.2807/1560-7917.es.2018.23.24.1700503.
- US Centers for Disease Control and Prevention. Whole genome sequencing. Atlanta, GA: US Department of Health and Human Services; 2020 [updated 2019 Dec 30; cited 2022 Aug 26]; Available from: https://www.cdc.gov/ncezid/dfwed/keyprograms/tracking-foodborne-illness-wgs.html.
- Petronella N, Ronholm J, Suresh M, Harlow J, Mykytczuk O, Corneau N, et al. Genetic characterization of norovirus GII.4 variants circulating in Canada using a metagenomic technique. BMC Infect Dis. 2018 Oct;18(1):1-11. Available from: https://bmcinfectdis.biomedcentral.com/articles/10.1186/s12879-018-3419-8.
- Silva AJ, Yang Z, Wolfe J, Hirneisen KA, Ruelle SB, Torres A, et al. Application of whole-genome sequencing for norovirus outbreak tracking and surveillance efforts in Orange County, CA. Food Microbiol. 2021 Sep;98:103796. Available from: https://doi.org/10.1016/j.fm.2021.103796.
- Allard MW, Strain E, Melka D, Bunning K, Musser SM, Brown EW, et al. Practical value of food pathogen traceability through building a whole-genome sequencing network and database. J Clin Microbiol. 2016 Aug;54(8):1975-83. Available from: https://doi.org/10.1128/jcm.00081-16.
- Crowe SJ, Green A, Hernandez K, Peralta VI, Bottichio L, Defibaugh-Chavez S, et al. Utility of combining whole genome sequencing with traditional investigational methods to solve foodborne outbreaks of salmonella infections associated with chicken: a new tool for tackling this challenging food vehicle. J Food Prot. 2017 Apr;80(4):654-60. Available from: https://doi.org/10.4315/0362-028x.jfp-16-364.
- US Food and Drug Administration. GenomeTrakr Network. Bethesda, MA: US FDA; [cited 2022 Aug 26]; Available from: https://www.fda.gov/food/whole-genome-sequencing-wgs-program/genometrakr-network.
- Doughman E. Whole genome sequencing: a new approach to food safety. WattPoultrycom. WattPoultry.com; 2021 [Dec]; Available from: https://www.wattagnet.com/articles/43870-whole-genome-sequencing-a-new-approach-to-food-safety.
- Bland RN, Johnson JD, Waite-Cusic JG, Weisberg AJ, Riutta ER, Chang JH, et al. Application of whole genome sequencing to understand diversity and presence of genes associated with sanitizer tolerance in listeria monocytogenes from produce handling sources. Foods. 2021 Oct;10(10):2454. Available from: https://doi.org/10.3390/foods10102454.
- Mi F, Hu C, Wang Y, Wang L, Peng F, Geng P, et al. Recent advancements in microfluidic chip biosensor detection of foodborne pathogenic bacteria: a review. Anal Bioanal Chem. 2022 Apr;414(9):2883-902. Available from: https://link.springer.com/article/10.1007/s00216-021-03872-w.
- Torre R, Costa-Rama E, Nouws HPA, Delerue-Matos C. Screen-printed electrode-based sensors for food spoilage control: bacteria and biogenic amines detection. Biosensors. 2020 Sep;10(10). Available from: https://doi.org/10.3390/bios10100139.
- Liu S, Poccia S, Candan KS, Chowell G, Sapino ML. epiDMS: data management and analytics for decision-making from epidemic spread simulation ensembles. J Infect Dis. 2016 Dec;214(suppl_4):S427-S32. Available from: https://doi.org/10.1093/infdis/jiw305.
- Oliver H, Abdo Z, Ricke S. Using WGS to protect public health (meeting summary). Washington, DC: Foundation for Meat & Poultry Research & Education; 2016. Available from: https://meatpoultryfoundation.org/sites/default/files/documents/WGS_Meeting_Summary.pdf.
- Petronella N, Kundra P, Auclair O, Hébert K, Rao M, Kingsley K, et al. Changes detected in the genome sequences of Escherichia coli, Listeria monocytogenes, Vibrio parahaemolyticus, and Salmonella enterica after serial subculturing. Can J Microbiol. 2019 Nov;65(11):842-50. Available from: https://doi.org/10.1139/cjm-2019-0235.
- Guo L, Ye L, Zhao Q, Ma Y, Yang J, Luo Y. Comparative study of MALDI-TOF MS and VITEK 2 in bacteria identification. J Thor Dis. 2014 May;6(5):534-8. Available from: https://doi.org/10.3978%2Fj.issn.2072-1439.2014.02.18.
- Acciari VA, Ruolo A, Torresi M, Ricci L, Pompei A, Marfoglia C, et al. Genetic diversity of Listeria monocytogenes strains contaminating food and food producing environment as single based sample in Italy (retrospective study). Int J Food Microbiol. 2022 Apr;366:109562. Available from: https://doi.org/10.1016/j.ijfoodmicro.2022.109562.
- Byrne L, Dallman TJ, Adams N, Mikhail AFW, McCarthy N, Jenkins C. Highly pathogenic clone of shiga toxin-producing Escherichia coli O157:H7, England and Wales. Emerg Infect Dis. 2018 Dec;24(12):2303-8. Available from: https://doi.org/10.3201%2Feid2412.180409.
- Carroll LM, Wiedmann M, Mukherjee M, Nicholas DC, Mingle LA, Dumas NB, et al. Characterization of emetic and diarrheal bacillus cereus strains from a 2016 foodborne outbreak using whole-genome sequencing: addressing the microbiological, epidemiological, and bioinformatic challenges. Front Microbiol. 2019 Feb;10. Available from: https://doi.org/10.3389/fmicb.2019.00144.
- Ford L, Ingle D, Glass K, Veitch M, Williamson DA, Harlock M, et al. Whole-genome sequencing of salmonella Mississippi and typhimurium definitive type 160, Australia and New Zealand. Emerg Infect Dis. 2019 Sep;25(9):1690-7. Available from: https://doi.org/10.3201%2Feid2509.181811.
- Gymoese P, Sørensen G, Litrup E, Elmerdal Olsen J, Møller Nielsen E, Torpdahl M, et al. Investigation of outbreaks of salmonella enterica serovar typhimurium and its monophasic variants using whole-genome sequencing, Denmark. Emerg Infect Dis. 2017 Oct;23(10):1631-9. Available from: https://doi.org/10.3201%2Feid2310.161248.
- Halbedel S, Wilking H, Holzer A, Kleta S, Fischer MA, Lüth S, et al. Large nationwide outbreak of invasive listeriosis associated with blood sausage, Germany, 2018-2019. Emerg Infect Dis. 2020 Jul;26(7):1456-64. Available from: https://doi.org/10.3201%2Feid2607.200225.
- Huth P, Wirth SE, Baker D, Nicholas DC, Douris A, Freiman J, et al. Ability of whole-genome sequencing to refine a salmonella I 4,[5],12:i:-cluster in New York state and detect a multistate outbreak linked to raw poultry. Food Prot Trends. 2021 Mar;41(2):239-45. Available from: https://www.foodprotection.org/publications/food-protection-trends/archive/2021-03-ability-of-whole-genome-sequencing-to-refine-a-salmonella-i-4-5-12-i-cluster-in-new-york-sta/.
- Joensen KG, Kiil K, Gantzhorn MR, Nauerby B, Engberg J, Holt HM, et al. Whole-genome sequencing to detect numerous campylobacter jejuni outbreaks and match patient isolates to sources, Denmark, 2015-2017. Emerg Infect Dis. 2020 Mar;26(3):523-32. Available from: https://doi.org/10.3201/eid2603.190947.
- Lachmann R, Halbedel S, Adler M, Becker N, Allerberger F, Holzer A, et al. Nationwide outbreak of invasive listeriosis associated with consumption of meat products in health care facilities, Germany, 2014–2019. Clin Microbiol Infect. 2021 Jul;27(7):1035. Available from: https://doi.org/10.1016/j.cmi.2020.09.020.
- Min J, Feng Z, Chao Y, Yinhua D, Kwan PSL, Yinghui L, et al. Whole-genome analysis of salmonella enterica serovar enteritidis isolates in outbreak linked to online food delivery, Shenzhen, China, 2018. Emerg Infect Dis. 2020 Apr;26(4):789-92. Available from: https://doi.org/10.3201/eid2604.191446.
- Moura A, Tourdjman M, Leclercq A, Hamelin E, Laurent E, Fredriksen N, et al. Real-time whole-genome sequencing for surveillance of listeria monocytogenes, France. Emerg Infect Dis. 2017 Sep;23(9):1462-70. Available from: https://doi.org/10.3201/eid2309.170336.
- Olufon O, Seale AC, Iyanger N, Wynne-Evans E. Use of whole-genome sequencing for public health intervention: outbreak investigation of a cluster of cases of salmonella foodborne illness in England, 2016. The Lancet. 2018 Nov;392(Supplement 2):S10-S. Available from: https://doi.org/10.1016/S0140-6736(18)32081-6.
- Simon S, Trost E, Bender J, Fuchs S, Malorny B, Rabsch W, et al. Evaluation of WGS based approaches for investigating a food-borne outbreak caused by Salmonella enterica serovar Derby in Germany. Food Microbiol. 2018 May;71:46-54. Available from: https://doi.org/10.1016/j.fm.2017.08.017.
- Smith C, Griffiths A, Allison S, Hoyano D, Hoang L. Escherichia coli O103 outbreak associated with minced celery among hospitalized individuals in Victoria, British Columbia, 2021. Can Commun Dis Rep. 2022 Jan;48(1):46-50. Available from: https://doi.org/10.14745/ccdr.v48i01a07.
- Svahn AJ, Chang SL, Rockett RJ, Cliff OM, Wang Q, Arnott A, et al. Genome-wide networks reveal emergence of epidemic strains of Salmonella Enteritidis. Int J Infect Dis. 2022 Apr;117:65-73. Available from: https://doi.org/10.1016/j.ijid.2022.01.056.
- Tanguay F, Vrbova L, Anderson M, Whitfield Y, Macdonald L, Tschetter L, et al. Outbreak of reading in persons of Eastern Mediterranean origin in Canada, 2014-2015. Can Commun Dis Rep. 2017 Jan;43(1):14-20. Available from: https://doi.org/10.14745/ccdr.v43i01a03.
- Vaughn EL, Vo QT, Vostok J, Stiles T, Lang A, Brown CM, et al. Linking epidemiology and whole-genome sequencing to investigate salmonella outbreak, Massachusetts, USA, 2018. Emerg Infect Dis. 2020 Jul;26(7):1538-41. Available from: https://doi.org/10.3201/eid2607.200048.
- Willi Q, Junior C-C, Orson M, Carmen VH, Maria LZ, Ronnie GG. Phylogenetic structure of Salmonella Enteritidis provides context for a foodborne outbreak in Peru. Sci Rep. 2020 Dec;10(1):1-6. Available from: https://www.nature.com/articles/s41598-020-78808-y.
- Integrated Rapid Infectious Disease Analysis (IRIDA). Highlights. Burnaby, BC: IRIDA; [cited 2022 Aug 26]; Available from: https://irida.ca/.
- US Centers for Disease Control and Prevention. PulseNet. Atlanta, GA: US CDC; [cited 2022 Aug 26]; Available from: https://www.cdc.gov/pulsenet/index.html.
- Boston Children's Hospital. HealthMap Foodborne Dashboard. Boston, MA: Boston Children's Hospital; [cited 2022 Aug 26]; Available from: https://www.healthmap.org/foodborne/.
Author
Dr. Ken Diplock
PhD, Public Health and Health Systems, University of Waterloo
Dr. Ken Diplock is the coordinator of the Bachelor of Environmental Public Health (BEPH) program at Conestoga College. Before joining Conestoga, Ken worked as a certified public health inspector with the Region of Waterloo Public Health and was actively involved in the Canadian Institute of Public Health inspectors (CIPHI).